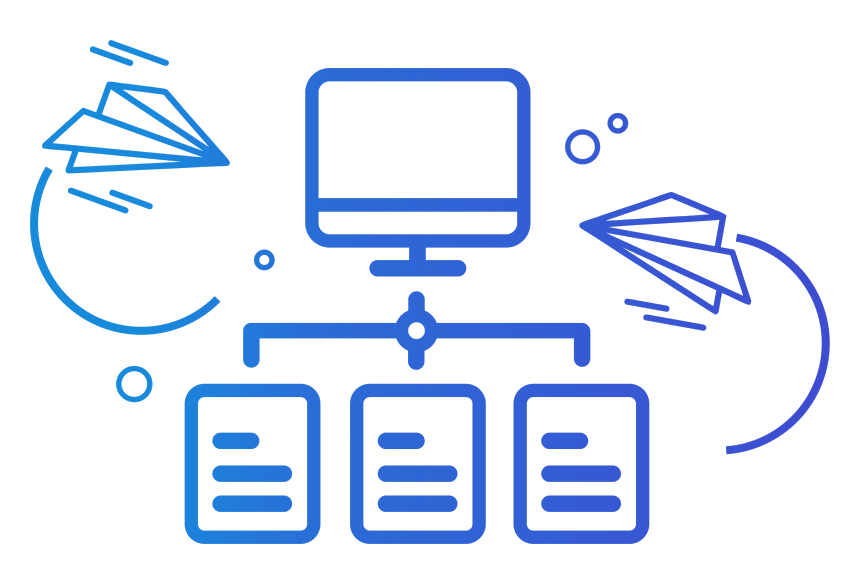
2010, Cloud-based GIS
Automated data scraping at large scales, creating maps never seen before
R, Python, Golang, Geoserver, and OGC-compliant GIS form the foundation of Ethos’ new era- Cloud based GIS
2014, New Insights from Advanced Geostatistics
The interrelations among data is the foundation of exploratory geoscience. Through analyzing geophysics, soil and rock geochemistry and structural patterns, we discovered- and continue to discover- underlying and fundamental principles in geology.
These principles are already leading to new mineral discoveries (see Idaho Copper Co), and exploration advancement for our clients.
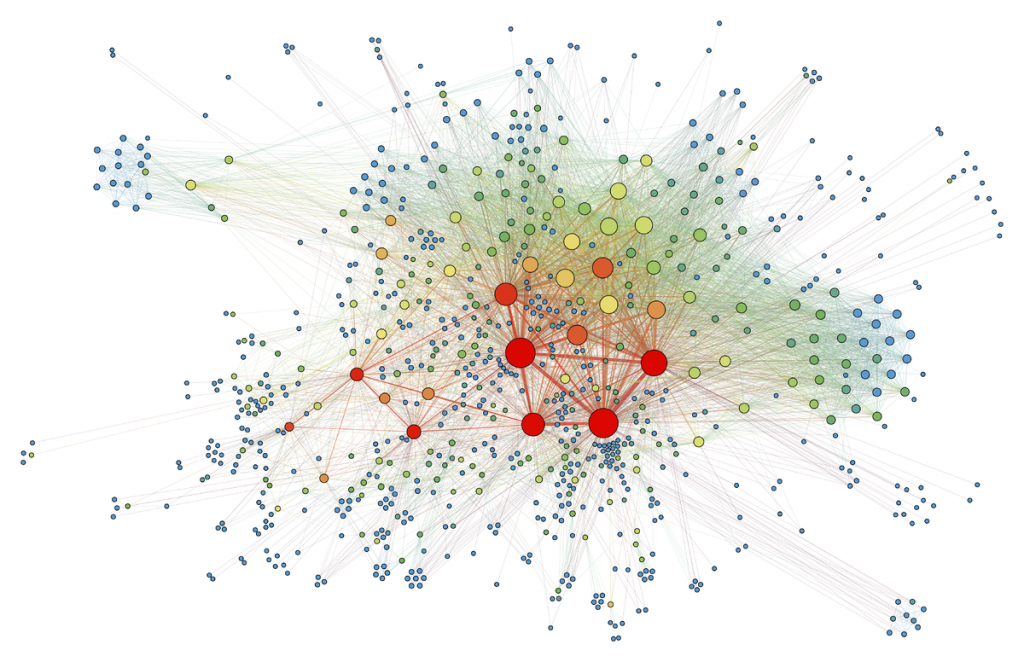
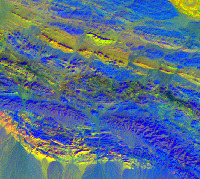
2019, Machine learning to create a mineral map of the world
Processing satellite hyperspectral data to search for mineral and geologic patterns in ROCKS, SOILS and VEGETATION.
Ethos started using K-nearest neighbors and supervised and unsupervised data analysis to build an automated processing utility that processes raw hyperspectral data and make giant mineral maps of the worlds surface.
The system exposes patterns in both soils and vegetation that relate to known and predicted geology, confirming the application of the method and the amazing opportunity to perform this work on a world-wide scale.
Today, leveraging these tools to enhance discovery for our clients
Data analysis and management should be fast, easy, and solve a problem. Our systems are built to be tools for the modern geologist that handle messy, real-world data to improve mineral targeting, generate success, and save money.
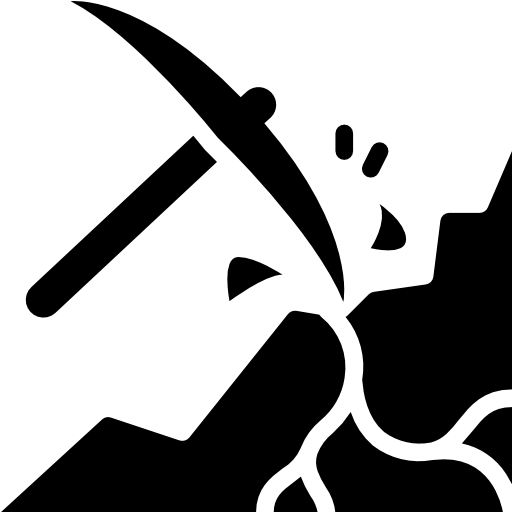